Dr. Adam Brandt of Stanford University’s Department of Energy Resources Engineering sent us a copy of a paper he wrote called Review of mathematical models of future oil supply: Historical overview and synthesizing critique. There seem to be quite a few things of interest, so I have summarized the paper, since the author did not have the time to do this. The box quotes are from the paper.
This paper has two goals. First, it provides a systematic review of oil depletion models produced to date. This serves to make obscure past works (often difficult to find) available to a wider audience so as to limit repetition of past efforts. Second, this paper provides synthesizing critique of previous modeling efforts, with the aim of improving future oil depletion modeling.
A major conclusion of the study is that existing models fare poorly at prediction:
Models based on quite disparate assumptions (e.g., physical simulation vs. economic optimal depletion) have produced approximately bell-shaped production profiles, but data do not support assertions that any one model type is most useful for forecasting future oil production. In fact, evidence suggests that existing models have fared poorly in predicting global oil production. The greatest promise for future developments in oil depletion modeling lies in simulation models that combine both physical and economic aspects of oil production.
Types of Models
Brandt describes four kinds of oil depletion models:
1. Curve Fitting Models
Curve-fitting models of oil production have been used since the 1950s. A variety of models exist, but their general approach is as follows.
1. Define a mathematical function to statistically fit to historical production data.
2. Include constraints to improve the quality of model fit.
3. Fit the constrained model to historical data to project future production.
Curve-fitting models vary in the function used, in the use of ultimately recoverable resources (URR) as a constraint and in the usage (or not) of symmetric model functions.
The paper provides descriptions of various curve fitting models, including Hubbert’s logistic model.
2. System simulations.
Simulation models (in our classification scheme) differ from curve-fitting models as follows: simulation models explicitly represent underlying physical and/or economic mechanisms that govern oil discovery and extraction, letting the shape of the production profile emerge from these mechanisms rather than specifying it in advance. These models include a broader range of independent variables, addressing a key problem of curve-fitting models noted by Taylor [38]: “No cause-and-effect relationship exists between time and the exploitation of crude oil.”
Thus, with the simulation models, one does not need to assume that the oil will be pumped out as quickly as possible. Oil production can follow demand, or some other approach. Brandt comments that a major difficulty of complex simulation models is the numerous input data that are required to parameterize the functional relationships. Figures 3 and 4 give an example of parts of a simulation model.
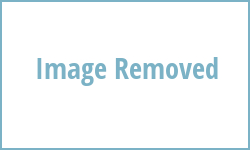
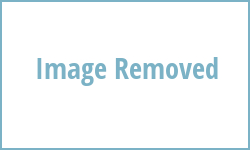
3. Bottom-up models
Bottom-up models use detailed field- or project-level data to “build up” projections of production from larger regions (such as a nation or the world). Bottom-up modeling has become increasingly prominent as discoveries have slowed and an increasing fraction of future oil is expected to come from already-discovered fields [52].
The most widely published bottom-up model is that of Campbell and co-authors, produced since the mid-1990s [53-55] using national-level models of production to generate global predictions. . .
Skrebowski has produced a bottom up model [58e61], using a database of oil field “megaprojects” e oil field development projects above a threshold size. Because large projects provide the majority of new oil output, this approach provides insight into short-term capacity increases.
4. Economic models of Oil Depletion
The major subcategories of these are
1. Economic optimal depletion theory, such as studied by Hotelling.
2. Economietic models of oil depletion, such as developed by Fisher and later by Kauffman and Cleveland.
Drawbacks and problems of existing models
1. Curve Fitting Models
Unfortunately, curve-fitting models are often used to make overly specific predictions of future production, ignoring many of the difficulties with such an approach.
An example is given by the excessive importance placed by some analysts on the supposed novelty and accuracy of Hubbert’s 1956 prediction. First, Hubbert’s prediction was predated by at least four publications that provided bell-shaped graphs of future U.S. oil production [13,14,81,82], and no fewer than 7 estimates from the 1950s predicted a peak in US oil production between 1963 and 1973, the approximate range of Hubbert’s low-high predictions (see Table 1). Second, Hubbert’s prediction of a peak in 1970 was based on his high value of URR, which he considered unlikely to be ach- ieved (and which itself was an underestimate). Interpretations of these facts vary: one could argue that Hubbert’s method was not extraordinary, as other methods also came close to predicting the peak date. . .
Another often neglected fact is that all of these studies of the 1950s underestimated URR, some significantly so, despite their reasonably correct projections of the peak date (see Table 1). Cumulative US production has already exceeded 200 Gbbl and significant reserves still remain. Thus, production has not dropped as quickly as Hubbert (or the other authors above) thought that it would, and the US curve is asymmetric [83].
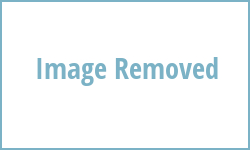
Major issues Brandt raises about curve fitting models include
a. Using exogenous estimates of URR to constrain curve-fitting models is problematic because estimates of URR have been too low in the past.
b. The use of logistic or bell-shaped functions is difficult to support with rigorous scientific reasoning.
c. Production profiles are often asymmetric, with slower rates of decline than rates of increase.
d. Curve fitting models do not account for economic factors, such as demand or resource substitution.
2. Simulation Models
a. Authors often make huge assumptions about the assumed functions and the parameters of the model.
b. Models are often unstable and finely balanced between positive and negative feedbacks. They may omit the role of inertia.
c. While models may fit past data well, they often have poor predictive powers.
3. Bottom’s up models
a. If a person builds a model from a magaprojects data base, or similar data base, the person needs more, rather than fewer assumptions about the process–peak production, decline rate, use of enhanced oil recovery
b. It is difficult to model projects not included, like infill drilling, workovers, and use of enhanced oil recovery.
c. The results are generally not reproducible; there is much reliance on the modeler’s judgment.
4. Economic Models
So far, they have been kept simple, but their predictive value has been low. They need to include political factors, but it is unclear how to include them.
The Problem of Prediction
Existing models have fared poorly in predicting global oil production. Even for models that are commonly thought to be successful, after-the-fact interpretation of the success or failure of a predictive effort is not easy (recall the discussion above of Hubbert’s successful prediction). . .
This author’s judgment with respect to the predictive value of models is as follows (noting that these topics are the source of much current debate):
1. Simple curve-fitting models can provide a first-order understanding of future production, assuming a given level of URR and no significant shocks to the system (e.g., demand continues to grow at rates within historical ranges). Such models are likely sufficient to predict the decade of peak production for an estimate of URR. The mathematical logic here is that consumption is so high during the years of peak production that minor variations in URR, or minor deviations due to political or economic factors, will not serve to significantly affect the date of the peak [111]. Unfortunately, such a conclusion is often of little practical use: major disruptions (e.g., the oil crises of the 1974 and 1979), or major errors in URR estimates have occurred in the past, and could occur again.
2. More-detailed mechanistic models (e.g., bottom-up, econometric), exhibit greater fidelity in reproducing historical data and are therefore likely more useful for near term predictions. But this advantage likely wanes for long-term forecasts because they are no less “brittle” with respect to uncertainties than other model types.
3. The most promising avenue for increasing our understanding of oil production lies in integrating the economic and physical factors of oil production.
4. There is no scientific justification for making specific predictions (e.g., the year of peak production) with any of the surveyed mathematical models: the uncertainties involved make such predictions of little use. Efforts should move away from making these kinds of predictions, and toward understanding the impacts of the inevitable transition to oil substitutes.
Improving Oil Depletion Modeling
It is no longer justifiable to build oil depletion models that neglect the reality of economic substitution with alternative resources like oil sands or coal-based liquid fuels. Nor should economic models neglect the underlying physical, geological, and engineering considerations that fundamentally drive the economics of oil production. Future progress will require building integrated models that account for both the economic and physical realities of oil production.